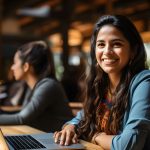
From Data to Decisions: Alternative Data Sources supporting SME Lending
From Data to Decisions: Alternative Data Sources supporting SME Lending Small and medium enterprises (SMEs) and microbusinesses are the backbone of many emerging and developing
At its core, lending is about trust. Who can lenders trust to repay a loan? Who can borrowers trust to offer fair terms?
The ways of working out who to trust in the world of credit have changed with technology and necessity over the years. Here is a short of overview of the history of credit scores.
For most of modern economic history, there was no such thing as a credit score. Who did, or did not, receive credit was determined at an individual level. The question of ‘trust’ and ‘character’ has been tied to lending for as long as people have borrowed.
Centuries ago, lenders only extended credit to business owners after asking around about them, gathering information from their neighbours and communities; they were essentially looking for character references.
The earliest credit reporting organisations were often small, local groups of merchants who would act as a collective to share experiences, helping them to avoid ‘high-risk’ individuals.
In 1770, the “Society of Guardians for the Protection of Trade Against Swindlers and Sharpers” was founded in London to collect information about individuals’ business and credit history. By the 1800s they began publishing this information in the form of the “City of London Trade Circular”.
In the USA, The Mercantile Agency is recognised as one of the first organised credit reporting agency, founded in 1841 following the economic recession of the late 1830s. It sought to make credit more reliable by collecting data on businessmen around the country. The agency had a network of correspondents that would seek answers to questions such as:
“Is he a man of fair character and good business habits?”
“Was he educated to merchandise?”
Firms could access this information through a subscription to the Mercantile Agency, requesting information on prospective customers, borrowers or business partners as to their honesty and reliability. The concept was a success and spread around the states, by the 1870s there were more than 10,000 correspondents around the country
These early character-based credit reference enjoyed wide-spread adoption in rapidly growing economies around the globe. However, critics highlighted the subjective nature of these reports.
With the rise of mass retail in the 1900s, these early ideas evolved into commercial bureaus that would collect information from department stores, shopkeepers and other vendors. Retailers would share information about their consumers, and access credit reports to assess who would pay back credit. This was combined with information collected included bankruptcies, divorces, lawsuits and arrests.
In the 1960s, there were more than 2,000 of these bureaus across the US.
This changed with the dawn of the computer age. The ability to digitise massive volumes of data and apply mathematical formulas to generate scores gave rise to a new era of credit.
Bill Fair and Earl Isaac were two mathematicians who worked to automate and standardise credit scoring. They founded Fair, Isaac and Company, eventually launching what we know today as the FICO score.
This has been the predominant method of evaluating who does, and does not, deserve a loan for the last three decades. This FICO 3-digit score was introduced in 1989, and, according to FICO itself, the algorithm and formula has not changed significantly since its introduction.
Today the bureau credit score is widely used to make lending decisions in developed markets across the world, but the scores are plagued by problems due to inaccurate or incomplete data. At launch FICO was touted to be a ‘universal’ credit score, however the reality is far from that. It has distilled credit worthiness to a small selection of data points, and in doing so excludes millions.
While the exact models vary, broadly speaking a bureau considers credit repayment history in the last five to seven years.
Today it is widely acknowledged that bureau credit scores reinforce the very biases that they were meant to eliminate. Applicants who do not have the specific data required are invisible to lenders relying on credit scores.
Thin-file and no-file customers (those with little to no credit history) are ‘invisible’ to financial institutions. A thin-file customer could be someone who is young, has recently moved to a new country, hasn’t used credit for a period of time (eg retirees who no longer have mortgages), or someone who generally pays with cash/debit cards rather than a credit card.
In 2022 the Federal Reserve estimated that one in 10 Americans are credit invisible[4], with marginalised communities disproportionately affected. According to Reuters, the number of Britons with poor credit ratings is rising in the wake of the COVID-19 pandemic, opening the door for increased predatory lending.
Without traditional credit history, these people won’t have a good credit score and financial institutions have no way of assessing risk. Traditional bureau scores have little flexibility and are not serving the needs of consumers and the wider community.
Increasingly lenders today are looking to diversify the data on which they rely for risk assessment and credit scoring. The availability of alternative data, supported by advanced AI and ML techniques, has proven to be predictive of risk while also allowing for credit inclusion for millions more people.
People are diverse, and credit assessment should consider a diversity of data. Non-traditional data, such as transaction data, open-banking data, smart-phone data and psychometric assessment has been proven to provide insight around the consumer’s personality, behaviour, community, skills and experience. Information which is highly predictive of creditworthiness.
Once a consumer opts-in, machine learning algorithms analyse thousands of data points for each application. Once the results are in, they can be incorporated in the lenders existing decisioning system.
Character-based insights can be used to enhance existing credit assessment processes with alternative data that is secure, privacy consented, real time, resilient and above-all, predictive.
The democratisation of data has put the power back in the hands of the consumer to choose when and how to leverage their own data to achieve their goals and access the services and opportunities meaningful to them.
For most of recorded history, people relied on character to determine who to trust with credit, but when this was up to the judgement of any one individual, there was always the risk of subjectivity affecting the outcome. Today, alternative data allows lenders to again include character through an objective approach. And allows borrowers to opt-in to sharing their data to be included in access to credit.
Photo by The New York Public Library on Unsplash
Read more from our blog
From Data to Decisions: Alternative Data Sources supporting SME Lending Small and medium enterprises (SMEs) and microbusinesses are the backbone of many emerging and developing